The integration of Artificial Intelligence (AI) in video surveillance technologies is revolutionizing the way organizations monitor, analyze, and respond to security threats. With advancements in machine learning and computer vision, AI enhances traditional surveillance systems, making them not only smarter but also significantly more effective. Explores the key AI technologies in video surveillance, including machine learning algorithms, deep learning for object detection, facial recognition, behavioral analysis, and anomaly detection in video streams.
The global AI in video surveillance market is expected to be valued at USD 5.6 billion in 2023 and is projected to reach USD 16.3 billion by 2028; it is expected to grow at a CAGR of 23.7% from 2023 to 2028. The advent of cloud computing has significantly impacted the AI capabilities in video surveillance. Cloud-based platforms provide scalable storage and computing resources, allowing for centralized management, remote access, and the integration of AI-powered analytics across multiple cameras and locations. These technological advancements have propelled the capabilities of AI in video surveillance, enabling more intelligent, efficient, and reliable security systems.
AI in Video Surveillance Technologies include :
Machine Learning Algorithms in Video Surveillance
Overview
Machine learning (ML) algorithms form the backbone of many advancements in video surveillance technology. These algorithms enable systems to learn from historical data, recognize patterns, and make predictions without needing explicit programming for each task. This adaptive learning capability is crucial for modern surveillance systems that must process large volumes of video data in real time.
Applications
- Real-time Monitoring: One of the most significant advantages of ML algorithms is their ability to process vast amounts of video data in real-time. This enables security personnel to receive alerts based on learned patterns and behaviors, allowing for timely responses to potential threats. For instance, an ML algorithm can monitor a busy retail store and immediately flag unusual movements, such as someone attempting to conceal an item.
- Event Classification: ML can classify events by analyzing behavior patterns, allowing the system to differentiate between normal activities and potential security threats. For example, it can recognize the difference between typical pedestrian movement and unusual behavior, such as someone loitering in a restricted area. This classification is critical in environments like airports or stadiums, where rapid identification of suspicious behavior is essential for safety.
Challenges
While ML provides significant advantages, challenges remain, such as ensuring data quality and the need for extensive training datasets. The effectiveness of ML algorithms heavily relies on the quality of the input data, which means organizations must invest in proper data collection and labeling processes. Additionally, the models must be regularly updated to adapt to new behaviors and environmental changes, which can be resource-intensive.
Deep Learning for Object Detection and Recognition in AI in Video Surveillance Technologies
Overview
Deep learning, a subset of machine learning, employs artificial neural networks with multiple layers to analyze and interpret complex data. This technology has significantly advanced the field of video surveillance, particularly in the areas of object detection and recognition.
Applications
- Object Detection: Deep learning algorithms excel at identifying and tracking objects within video feeds, such as people, vehicles, and other items of interest. This capability is vital for applications such as monitoring entry points in secure facilities or tracking movements in a retail environment. By analyzing each frame of video in real time, these systems can recognize specific objects and follow their movements, providing valuable insights into potential security breaches.
- Facial Recognition: Deep learning has greatly improved the accuracy of facial recognition systems, which are increasingly used in security applications. These systems can identify individuals in real time by comparing facial features against databases of known individuals. This capability is particularly valuable for law enforcement and security agencies, enabling quick identification of suspects or missing persons, thereby enhancing public safety.
Advantages
The primary advantage of deep learning in video surveillance is its high accuracy and robustness in diverse environmental conditions, such as varying lighting and angles. Unlike traditional algorithms, deep learning can learn complex patterns and features directly from the data, which allows for greater adaptability. However, it requires substantial computational resources and well-curated datasets for effective training, which may present challenges for smaller organizations.
Download PDF Brochure @ https://www.marketsandmarkets.com/pdfdownloadNew.asp?id=84216922
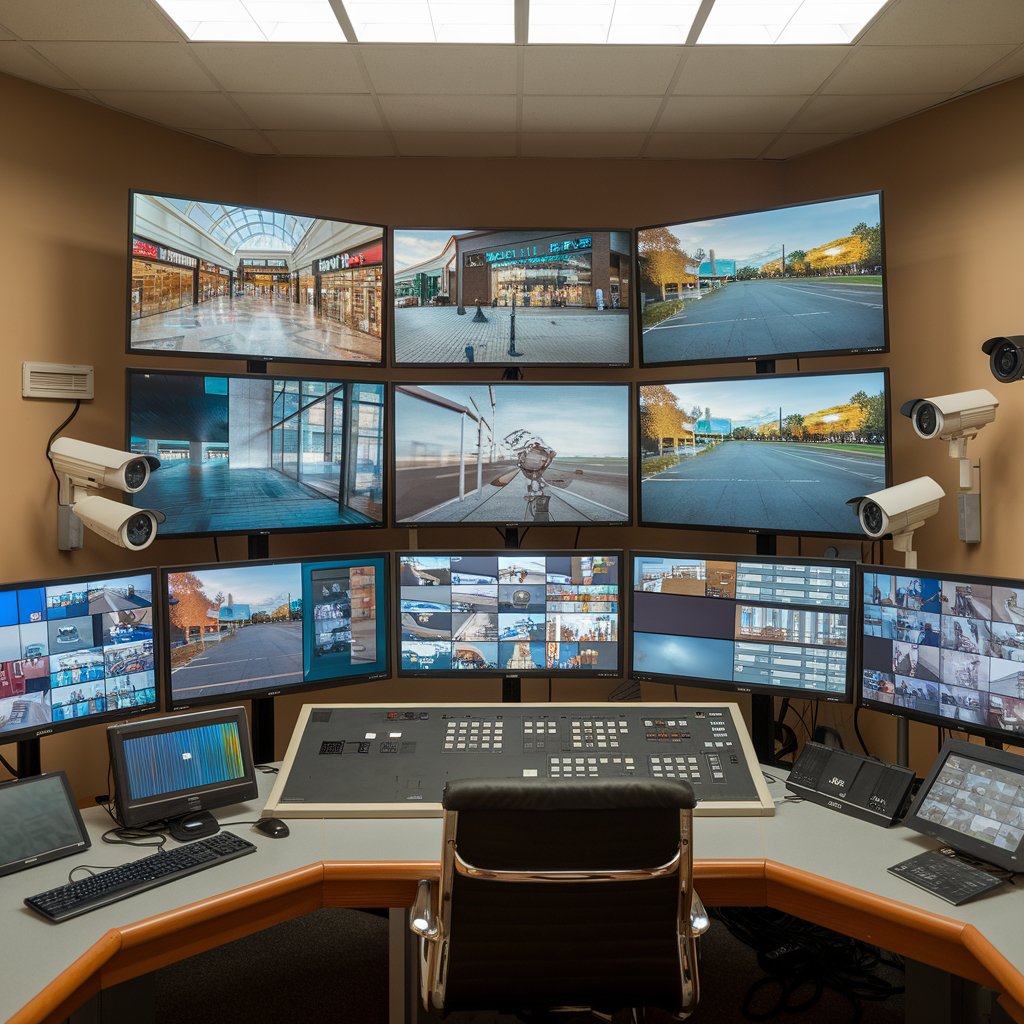
AI in Video Surveillance Technologies : Facial Recognition Technologies
Overview
Facial recognition technologies have gained significant traction in video surveillance, enabling systems to identify and verify individuals based on facial features. This technology is increasingly being integrated into security systems across various sectors.
Applications
- Security and Access Control: Facial recognition systems are widely used for security applications, particularly for access control in restricted areas. By using facial recognition, organizations can ensure that only authorized personnel can enter sensitive areas, such as data centers or secure offices. This method reduces reliance on physical ID cards, which can be lost or stolen, thereby enhancing overall security.
- Law Enforcement: Law enforcement agencies utilize facial recognition to identify suspects in real time, aiding in investigations and improving public safety. By analyzing surveillance footage from public spaces, these systems can quickly match faces against databases of known offenders, facilitating rapid responses to potential threats.
Challenges
Despite its advantages, facial recognition technology in AI in Video Surveillance Technologies faces several challenges. Privacy concerns are at the forefront, as individuals may feel uncomfortable with constant surveillance and data collection. Additionally, the accuracy of facial recognition systems can be affected by environmental factors, such as poor lighting or occlusions (like masks or hats). There are also concerns about algorithmic biases, which can lead to higher false identification rates for certain demographic groups. Addressing these issues is crucial for broader acceptance and responsible deployment of this technology.
Behavioral Analysis using AI
Overview
AI-powered behavioral analysis involves monitoring and analyzing human behavior in video feeds to detect unusual or suspicious activities. This AI in video surveillance application enhances situational awareness and helps security personnel respond more effectively to potential threats.
Applications
- Suspicious Behavior Detection: AI algorithms can analyze video footage to identify behavioral patterns associated with potential threats. For instance, they can flag aggressive movements or prolonged loitering in restricted areas. This capability allows security teams to intervene before a situation escalates, enhancing safety in public spaces like airports, shopping malls, and large events.
- Crowd Behavior Analysis: In urban environments, AI can analyze crowd behavior to identify potential risks, such as panic or overcrowding. By monitoring video feeds from public events or transportation hubs, AI systems can provide alerts when crowd densities exceed safe levels, allowing authorities to manage situations proactively and ensure public safety.
Benefits
The benefits of behavioral analysis include enhanced situational awareness and improved response times to incidents. By focusing on behaviors indicative of threats, security personnel can prioritize their attention on high-risk areas. Additionally, this technology can aid in resource allocation, ensuring that security measures are deployed where they are needed most.
Anomaly Detection in Video Streams
Overview
Anomaly detection involves identifying patterns in video data that deviate from the norm, indicating potential security incidents. This capability is essential for maintaining a secure environment in various applications.
Applications
- Intrusion Detection: AI systems can monitor video feeds for unauthorized access or unusual activity, sending alerts to security personnel when anomalies are detected. For example, if an individual enters a secure area outside of normal operating hours, the system can trigger an immediate alert, allowing for a rapid response.
- Environmental Monitoring: Anomaly detection can also be applied to monitor environmental changes, such as unusual smoke, fire, or flooding. By analyzing video feeds from surveillance cameras, these systems can provide critical alerts for emergency situations, enabling quicker response times and potentially saving lives.
Advantages
The primary advantage of anomaly detection is its ability to provide real-time alerts for potential threats, which significantly reduces response times and enhances overall security measures. However, ensuring the reliability of these systems requires sophisticated algorithms to minimize false positives. Continuous training and adjustment of the models are essential to adapt to changing environments and behaviors, ensuring the system remains effective.
AI is significantly enhancing video surveillance technologies, transforming how we approach security and monitoring. Machine learning algorithms, deep learning for object detection and recognition, facial recognition technologies, behavioral analysis, and anomaly detection are just a few examples of how AI is reshaping the industry. These innovations not only improve the effectiveness of surveillance systems but also provide a proactive approach to security, enabling faster responses and better protection for individuals and assets. As AI continues to evolve, its integration into video surveillance will become increasingly critical, driving advancements that enhance safety and operational efficiency across various sectors. The future of AI in video surveillance is not just about passive monitoring; it’s about proactive engagement, intelligent analysis, and fostering safer environments for communities worldwide.