Neuromorphic computing is a revolutionary approach that emulates the structure and function of the human brain, providing an efficient, adaptive, and energy-conscious alternative to traditional computing methods. By mimicking the brain’s processes, neuromorphic systems are designed to solve complex problems with high performance and minimal energy consumption. This article highlights the numerous benefits of neuromorphic computing and its potential to shape the future of artificial intelligence (AI), robotics, autonomous systems, and beyond.
The neuromorphic computing market size is expected to grow from USD 28.5 million in 2024 and is estimated to reach USD 1,325.2 million by 2030; it is expected to grow at a Compound Annual Growth Rate (CAGR) of 89.7% from 2024 to 2030. Growth in the neuromorphic computing market is driven through the integration of neuromorphic computing in automotive and space operations. In space, where bandwidth is limited and the communication delay might be considered large, onboard processing capabilities are crucial. The neuromorphic processor analyzes and filters data at the point of collection, reducing the need to transmit large datasets back to Earth. whereas, in automobile sector, neuromorphic processors can make autonomous driving systems more responsive by onboard real-time processing with minimal latency so that safety is ensured along with efficiency.
Benefits of Neuromorphic Computing
1. Energy Efficiency
Neuromorphic computing significantly outperforms traditional computing systems in terms of energy consumption.
Traditional computing relies on centralized processing and constant data communication between the CPU and memory, which consumes substantial power. Neuromorphic systems, on the other hand, are designed to mimic the brain’s parallel processing capabilities, using localized data processing to minimize energy usage. The spiking neural networks (SNNs) that power these systems only activate neurons when necessary, reducing the power needed for computation.
This makes neuromorphic chips highly suited for battery-powered IoT devices, autonomous vehicles, and mobile devices, where power consumption is a critical concern. In fact, some neuromorphic systems can operate with orders of magnitude lower power than traditional processors, extending the lifespan of devices that rely on small batteries.
For instance, companies like Intel and IBM have developed neuromorphic chips such as Loihi and TrueNorth, demonstrating significant energy savings while executing AI tasks such as pattern recognition and real-time data processing.
2. Real-Time Processing and Adaptability
One of the standout features of neuromorphic computing is its ability to process data in real-time and quickly adapt to new inputs and environments.
Unlike traditional AI systems, which rely on centralized data processing and can suffer from delays, neuromorphic systems excel at reacting to stimuli and making decisions in milliseconds. This is achieved through their parallel, event-driven architecture, which mimics the brain’s ability to process sensory information immediately and adjust behaviors accordingly.
Such real-time decision-making is essential for applications like autonomous vehicles, where split-second reactions to environmental changes (e.g., avoiding an obstacle or adjusting speed) are crucial. In robotics, this capability is useful for tasks such as dynamic motion control and human-robot interaction, enabling robots to react to their environment without delay.
In military or industrial applications, neuromorphic systems can enhance surveillance or security systems by quickly analyzing sensor data and identifying patterns or threats in real-time. The faster data processing enables rapid action, such as activating alerts or autonomously adjusting operational parameters.
3. Enhanced Pattern Recognition and Learning
Neuromorphic systems excel at pattern recognition and unsupervised learning, making them particularly well-suited for tasks that require identifying complex patterns from noisy or incomplete data.
The brain’s ability to learn and recognize patterns from limited or fragmented information serves as the basis for neuromorphic computing’s strength. By mimicking this process, neuromorphic systems can detect patterns in data without the need for massive, structured datasets. This makes them more robust and adaptable than traditional machine learning models, which often require large amounts of labeled data to train effectively.
In applications such as speech recognition, image analysis, and natural language processing, neuromorphic systems can continuously learn from new inputs, improving their performance over time. This is particularly valuable in situations where data is sparse, inconsistent, or difficult to label, such as in medical diagnostics or predictive maintenance.
Download PDF Brochure @ https://www.marketsandmarkets.com/pdfdownloadNew.asp?id=227703024
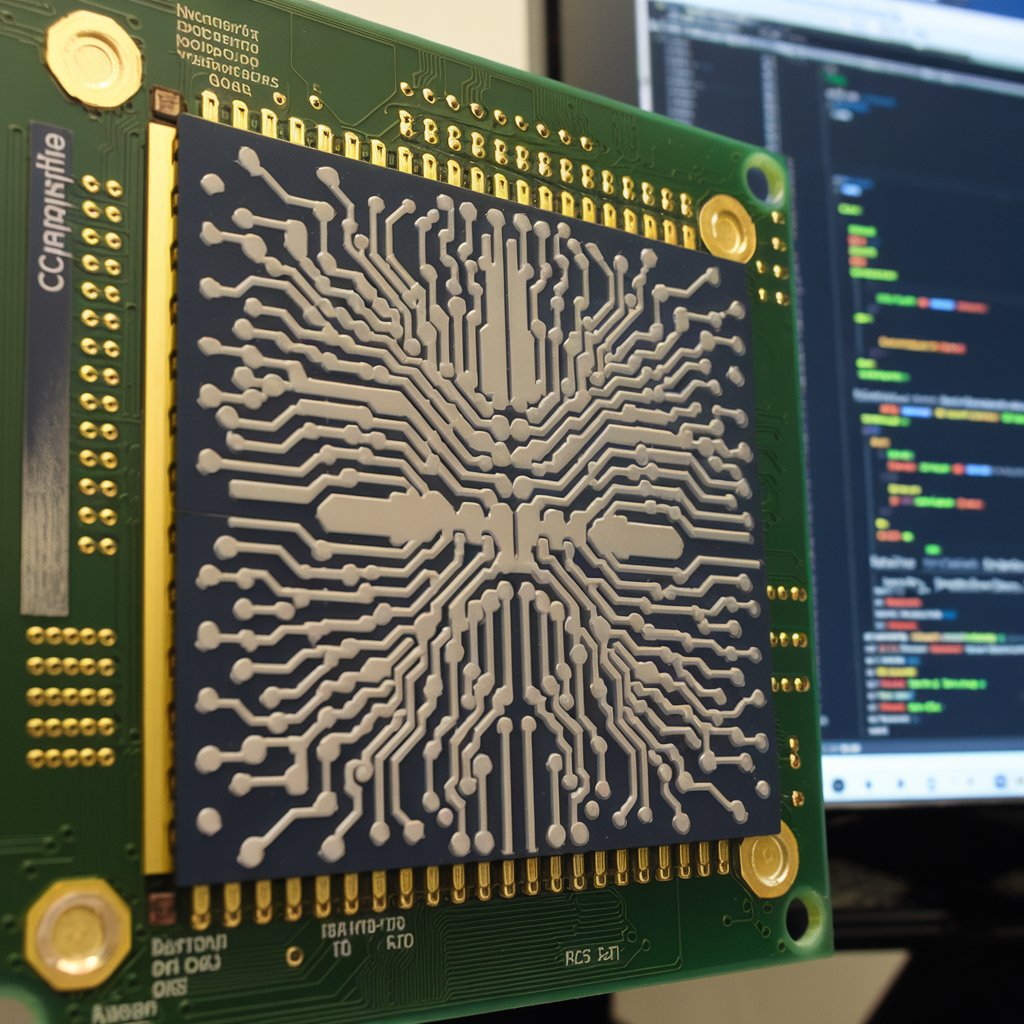
4. Scalability and Integration with Brain-Like AI
Neuromorphic systems are highly scalable, allowing them to grow in complexity as needed without compromising performance.
The modular nature of neuromorphic chips means that they can be expanded or connected in a manner similar to how the human brain uses interconnected neurons to process increasingly complex information. These scalable systems are ideal for AI applications that need to process vast amounts of data or adapt to growing tasks over time.
As neuromorphic computing integrates with other AI paradigms like deep learning and reinforcement learning, it offers the potential for more powerful, self-learning systems. These systems can improve performance based on experience, much like how humans learn over time by observing the world around them.
This level of adaptability and scalability is useful for creating autonomous systems such as drones, smart cities, and personalized healthcare technologies. These systems can evolve and optimize themselves based on real-world data, leading to more efficient and intelligent behavior.
5. Reduced Latency and Improved Efficiency in Edge Computing
Neuromorphic computing’s distributed processing capability makes it a perfect fit for edge computing applications that require fast, localized decision-making.
Traditional AI models rely heavily on cloud computing, which can introduce latency due to the need to send data to centralized servers for processing. Neuromorphic systems, however, are capable of handling computation locally at the edge of the network, reducing the time it takes to make decisions. This is particularly important in real-time systems where every millisecond counts, such as in autonomous vehicles, smart cameras, and industrial sensors.
By processing data closer to the source, edge devices using neuromorphic computing reduce the need for constant communication with a central server, lowering bandwidth demands and minimizing delays. This not only improves efficiency but also ensures that critical decisions are made instantaneously, even in remote or bandwidth-limited environments.
For instance, smart city infrastructure, such as traffic lights or public safety systems, can benefit from neuromorphic chips that analyze sensor data locally and make real-time adjustments to traffic flow or security monitoring without waiting for cloud processing.
6. Robustness to Faults and Errors
Neuromorphic computing industry offers a significant advantage in terms of fault tolerance and resilience to system failures.
Because neuromorphic systems are based on a decentralized architecture, they are inherently more resilient to hardware failures. In a traditional system, if one part of the processor fails, it can disrupt the entire operation. However, in neuromorphic systems, the failure of individual neurons or components often does not stop the system from functioning, as other neurons can compensate for the loss. This self-healing capability makes neuromorphic systems ideal for mission-critical applications such as space exploration, military defense, and medical devices, where reliability is paramount.
For example, in a space mission, where equipment is subject to harsh conditions and communication may be limited, neuromorphic computing could ensure that spacecraft or rovers continue to operate efficiently, even if certain components fail or experience performance degradation.
The Future of Neuromorphic Computing
Neuromorphic computing is opening up new possibilities for AI and machine learning, with its energy efficiency, real-time decision-making, and adaptive learning capabilities. As more industries adopt neuromorphic technologies, we can expect breakthroughs in sectors such as autonomous systems, robotics, edge computing, healthcare, and smart devices.
The ability to process information in parallel, reduce latency, and learn from experience positions neuromorphic computing as the ideal architecture for next-generation AI applications. As research and development continue to evolve, neuromorphic systems will become even more powerful, paving the way for intelligent, self-optimizing technologies that are more efficient and adaptable than ever before.
In the coming years, neuromorphic computing is set to redefine how we interact with machines, offering an unprecedented level of intelligence and efficiency for both consumer and industrial applications
Key companies operating in the neuromorphic computing companies are :
- Intel Corporation (US),
- IBM (US),
- Qualcomm Technologies, Inc. (US),
- Samsung Electronics Co., Ltd. (South Korea),
- Sony Corporation (Japan),
- BrainChip, Inc. (Australia),
- SynSense (China),
- MediaTek Inc. (Taiwan),
- NXP Semiconductors (Netherlands),
- Advanced Micro Devices, Inc. (US),
- Hewlett Packard Enterprise Development LP (US),
- OMNIVISION (US), among others.