Artificial Intelligence (AI) is transforming industries across the globe, and manufacturing is no exception. AI-based fault detection and classification systems are revolutionizing the way manufacturers identify, diagnose, and prevent issues on the production line. By leveraging machine learning (ML), data analytics, and sensor integration, AI-driven systems provide powerful tools to improve product quality, reduce waste, and optimize production efficiency. Here’s how AI is making an impact on fault detection and classification in manufacturing:
The global fault detection and classification Industry was valued at USD 4.4 billion in 2022 and is projected to reach USD 7.4 billion by 2028; it is expected to register a CAGR of 8.9% between 2023 and 2028 The rise in demand for FDC systems is attributed to the increased complexity of systems, strong focus of manufacturers on automating quality control and quality assurance processes, and stringent health and safety measures imposed by governments and standards organizations on global manufacturing firms.
Impact of AI in Fault Detection and Classification in Manufacturing
1. Early Error Detection: Preventing Defective Parts from Moving Down the Production Line
One of the most significant advantages of AI in fault detection is its ability to detect errors early in the manufacturing process, often before they become visible to the human eye. AI-powered systems use sensors (such as vision systems, temperature sensors, and vibration sensors) combined with machine learning algorithms to monitor real-time data from the production line. By continuously analyzing these data streams, AI can identify anomalies and deviations from the desired operational parameters.
For instance, in a complex assembly line, if a part is incorrectly assembled or a machine is operating outside its optimal range, AI can immediately flag the issue and alert operators or automatically halt production to prevent defective parts from moving further down the line. This early detection not only prevents faulty products from reaching customers but also reduces the need for costly rework and waste.
2. Increased Production Volume Without Sacrificing Quality
AI’s ability to detect faults early and accurately means that manufacturers can maintain high production volumes while ensuring quality is never compromised. Traditional manual inspection processes are time-consuming and often prone to human error, especially in high-speed environments. AI-based fault detection systems, on the other hand, can work 24/7 with high consistency and precision, leading to higher throughput without sacrificing product quality.
For example, AI-driven vision systems can inspect every part of a product as it moves along the assembly line, identifying defects such as surface blemishes, incorrect assembly, or dimensional inaccuracies. Since AI operates faster and more accurately than manual inspection, manufacturers can increase production rates while maintaining stringent quality control standards.
3. Tracking Historical Data to Pinpoint Issues and Improve Future Production Processes
AI-powered fault detection systems don’t just work in real-time; they also provide valuable insights from historical data. By continuously collecting data from sensors and production equipment, AI can track performance over time and identify recurring patterns or trends that may indicate underlying issues. This historical analysis allows manufacturers to pinpoint the root causes of recurring faults and take proactive measures to prevent them in the future.
For example, if a particular machine experiences consistent vibrations that lead to failures after a certain number of cycles, AI can analyze past performance data to identify when and why the failure occurs. By predicting when the machine is likely to fail, AI helps manufacturers schedule preventative maintenance, optimize production schedules, and avoid unplanned downtime. The ability to track and analyze historical data also enables continuous improvement in manufacturing processes, allowing companies to refine their operations and reduce defects over time.
Download PDF Brochure @ https://www.marketsandmarkets.com/pdfdownloadNew.asp?id=15954762
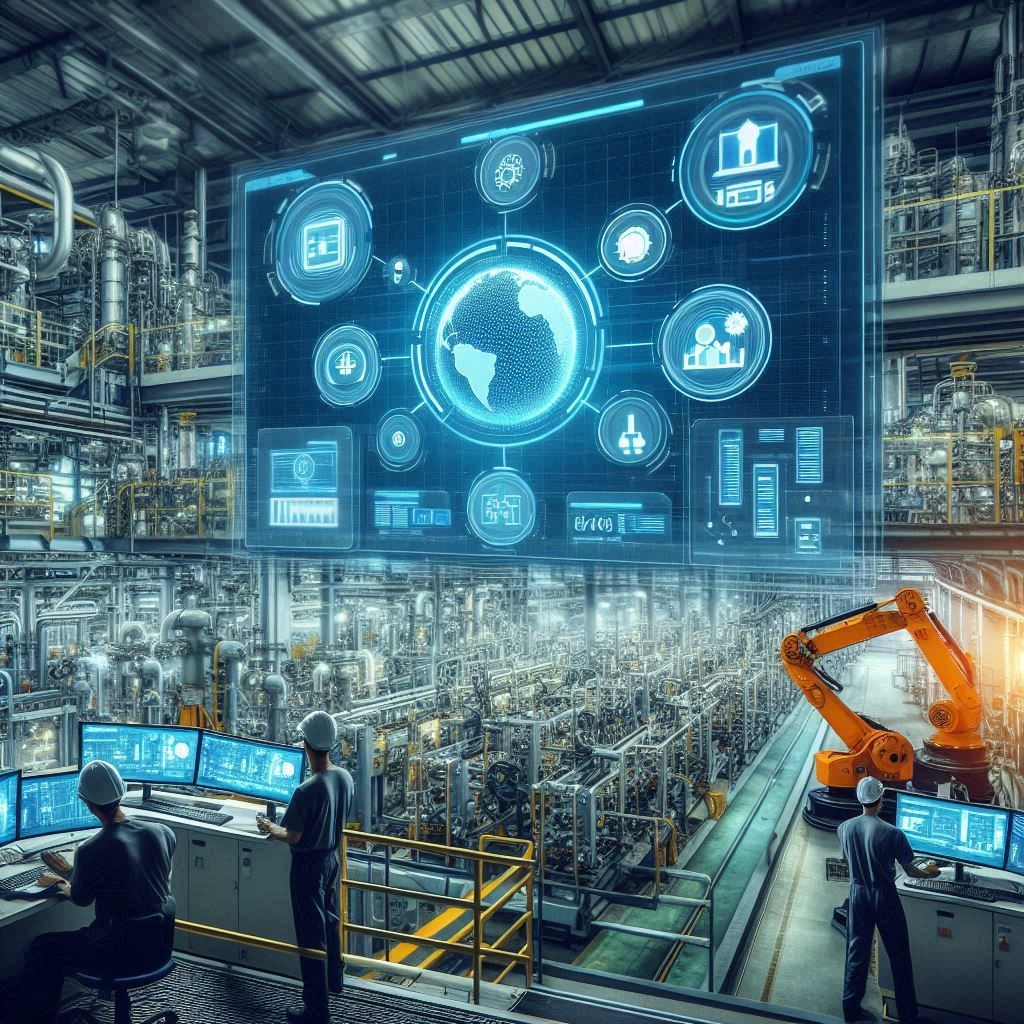
4. Optimized Incoming Material Inspection
AI isn’t just useful for monitoring the production process—it can also enhance the quality control of raw materials entering the manufacturing facility. Materials that are defective or not up to specification can lead to production delays, poor-quality products, and unnecessary waste. AI-based systems can optimize the incoming material inspection process by automating the detection of defects and inconsistencies in raw materials before they are even used in production.
For instance, AI-powered image recognition systems can scan incoming materials (such as metal sheets, plastic components, or electronic parts) for defects like cracks, surface imperfections, or dimensional inaccuracies. This enables manufacturers to identify subpar materials before they are incorporated into the production process, reducing the likelihood of faults downstream. Moreover, AI can be trained to recognize patterns in material-related failures, helping to source higher-quality materials and improve the overall supply chain.
5. Reaching, and Often Surpassing, Human-Level Accuracy
One of the most compelling aspects of AI in fault detection and classification is its potential to reach, and even surpass, human-level accuracy in certain tasks. While human inspectors may miss subtle defects due to fatigue, distraction, or limited capacity for processing large amounts of data, AI systems excel at analyzing vast quantities of data with speed and precision. This leads to improved accuracy in fault detection, particularly in complex systems where faults may not be immediately visible or easily identifiable.
Machine learning algorithms, particularly those in deep learning, can be trained to recognize even the most subtle anomalies in manufacturing processes. For instance, AI models can analyze patterns in vibration signals to detect early signs of mechanical failure, or they can detect very fine defects in images of products, such as micro-cracks in semiconductor components. As AI continues to improve, it is not only matching but often exceeding human-level detection capabilities, leading to fewer defects and more consistent product quality.
Additional Benefits of AI-Based Fault Detection and Classification
a. Real-Time Monitoring and Actionable Insights
AI-based systems can provide manufacturers with real-time visibility into production processes, enabling faster decision-making and immediate corrective actions when faults are detected. Whether through automated alerts or direct intervention in the manufacturing process, AI allows for rapid response times that help minimize disruptions and prevent larger issues from developing.
b. Cost Savings and ROI
While implementing AI in fault detection requires an initial investment in sensors, software, and training, the long-term cost savings can be substantial. By reducing defect rates, minimizing waste, and avoiding unplanned downtime, manufacturers can see a significant return on investment (ROI). Moreover, predictive maintenance powered by AI reduces the costs associated with unexpected equipment failures and ensures that resources are used more efficiently.
c. Enhanced Safety and Compliance
AI can improve safety on the factory floor by detecting hazardous conditions or equipment malfunctions before they lead to accidents. For example, AI can monitor temperature, pressure, and vibration levels on machines to identify dangerous conditions that could cause equipment failure or injury. Additionally, AI can help ensure compliance with industry regulations by monitoring processes and flagging deviations that could lead to non-compliance.
AI-based fault detection and classification systems are changing the landscape of manufacturing, offering powerful tools to detect and address issues early, increase production capacity, and maintain high-quality standards. From preventing defective parts from moving down the line to optimizing material inspections and tracking historical data for continuous improvement, AI provides manufacturers with the means to optimize every aspect of the production process.
As AI technology continues to evolve, its capabilities in fault detection will only expand, providing even more opportunities for manufacturers to boost efficiency, reduce waste, and improve product quality. For manufacturers looking to stay competitive in the digital age, embracing AI-powered fault detection is no longer just an option—it’s a strategic necessity.
AI-based fault detection and classification companies are :
- Keyence Corporation (Japan),
- Cognex Corporation (US),
- KLA Corporation (US),
- Teledyne Technologies (US),
- OMRON Corporation (Japan),
- Microsoft (US),
- Tokyo Electron Limited (Japan),
- Siemens (Germany),
- Amazon Web Services, Inc. (US),
- Synopsys, Inc. (US),
- Applied Materials, Inc. (US),
- einnoSys Technologies Inc. (US),
- Datalogic(Italy),
- PDF Solutions (US),
- Nikon Corporation (Japan),
- INFICON (Switzerland),
- Qualitas Technologies. (India),
- BeyondMinds (Israel),
- elunic AG (Germany),
- Chooch Intelligence Technologies (US),
- KILI TECHNOLOGY (France),
- MobiDev (US),
- DWFritz Automation, LLC (US),
- Radiant Optronics Pte Ltd (Asia),
- Visionify. (US),
- SAMSUNG SDS (South Korea),
- LS ELECTRIC Co., Ltd. (South Korea),
- Doosan Corporation (South Korea), and
- Hyundai Heavy Industries (South Korea)
Frequently Asked Questions (FAQ) – Impact of AI in Fault Detection and Classification in Manufacturing
- How does AI improve fault detection and classification in manufacturing? AI improves fault detection and classification by analyzing large volumes of data in real-time, identifying patterns, and detecting anomalies that may indicate potential defects. AI algorithms can classify faults accurately, allowing manufacturers to take corrective action before defects impact production.
- What are the benefits of using AI in fault detection and classification? The key benefits include faster identification of defects, reduced downtime, improved product quality, higher production yields, and more efficient maintenance schedules. AI systems can detect even subtle issues that may be missed by traditional methods, leading to better overall process control.
- What types of faults can AI systems detect and classify? AI can detect a wide range of faults in manufacturing, such as equipment malfunctions, process deviations, material defects, and system inefficiencies. It can classify these faults into categories, helping operators understand the root cause and take appropriate action.
- How does AI handle real-time fault detection in manufacturing? AI-powered systems use machine learning algorithms to continuously monitor data from sensors, machines, and other sources. By analyzing this data in real-time, AI systems can quickly detect anomalies or deviations from normal operations, triggering alerts and enabling immediate corrective actions.
- What role does machine learning play in fault detection and classification? Machine learning (ML) allows AI systems to learn from historical data, improving their ability to predict and classify faults accurately. ML algorithms can continuously improve their performance by learning from new data, making them increasingly effective over time.