The field of Digital Signal Processing (DSP) is evolving rapidly, driven by the increasing need for more advanced, efficient, and versatile signal processing capabilities. Traditionally, DSPs have been integral in a wide range of applications— from audio and video processing to communications and sensor data analysis. However, with the emergence of new technologies, DSPs are taking on more complex tasks and enabling real-time processing that was previously thought to be beyond their reach.
In this blog, we’ll explore some of the top emerging technologies in the DSP industry, shedding light on how they are reshaping signal processing and opening up new possibilities for industries ranging from healthcare and telecommunications to automotive and entertainment.
Top Emerging Technologies in Digital Signal Processing (DSP) Included :
The Digital Signal Processor market size is projected to grow from USD 10.1 billion in 2024 and is projected to reach USD 14.7 billion by 2029; it is expected to grow at a CAGR of 7.8% from 2024 to 2029. The growth of the Digital Signal Processor market is driven by surging adoption of Internet of Things (IoT) and connected devices.
1. Artificial Intelligence (AI) and Machine Learning (ML) Integration
AI and machine learning (ML) are perhaps the most transformative forces driving the next wave of DSP innovation. By integrating AI algorithms into DSP architectures, these processors are becoming smarter and more capable of handling complex tasks in real time. Unlike traditional DSPs, which focus on well-defined mathematical operations, AI-enabled DSPs can process data dynamically, adapting to new information and improving performance over time.
For example, speech recognition, image enhancement, object detection, and predictive analytics are all made possible through AI-powered DSPs. This opens the door to applications such as:
- Autonomous vehicles, where AI-driven DSPs process sensor data in real time to make crucial decisions.
- Smart home devices that use voice recognition and natural language processing to understand user commands.
Benefit: AI-driven DSPs make signal processing smarter, more adaptive, and capable of learning from data, significantly improving the accuracy and efficiency of tasks like speech recognition and real-time image processing.
2. Edge Computing and Real-Time Signal Processing
The rise of edge computing is significantly affecting the DSP industry by bringing computation closer to the source of data. Instead of relying on cloud servers to process data, edge devices with integrated DSPs can now perform complex signal processing locally, reducing latency and enabling faster decision-making.
For instance, in IoT devices, wearables, and autonomous vehicles, real-time signal processing is essential for applications like sensor data interpretation and object detection. By processing data on the edge, DSPs ensure faster responses while optimizing network bandwidth usage.
Example: In smart cameras used for security or monitoring, edge DSPs can analyze video feeds locally, enabling instant object detection and decision-making without needing to send data to a centralized cloud.
Benefit: Edge computing with DSPs reduces latency, conserves bandwidth, and enhances the responsiveness of real-time applications.
3. Quantum Computing in DSPs
Though still in the early stages, quantum computing is poised to revolutionize DSP technology by enabling the processing of exponentially more complex signals. Quantum algorithms have the potential to perform tasks like Fourier transforms, matrix multiplication, and signal encryption much faster than classical DSPs. This could dramatically speed up signal processing tasks and open new doors for applications that require immense computational power.
Example: In applications like signal encryption and video compression, quantum computing could significantly enhance DSP performance by quickly processing massive datasets that would otherwise take much longer on traditional systems.
Benefit: Quantum computing in DSPs could dramatically improve processing speed and efficiency, especially for complex tasks that are computation-heavy.
Download PDF Brochure @ https://www.marketsandmarkets.com/pdfdownloadNew.asp?id=794
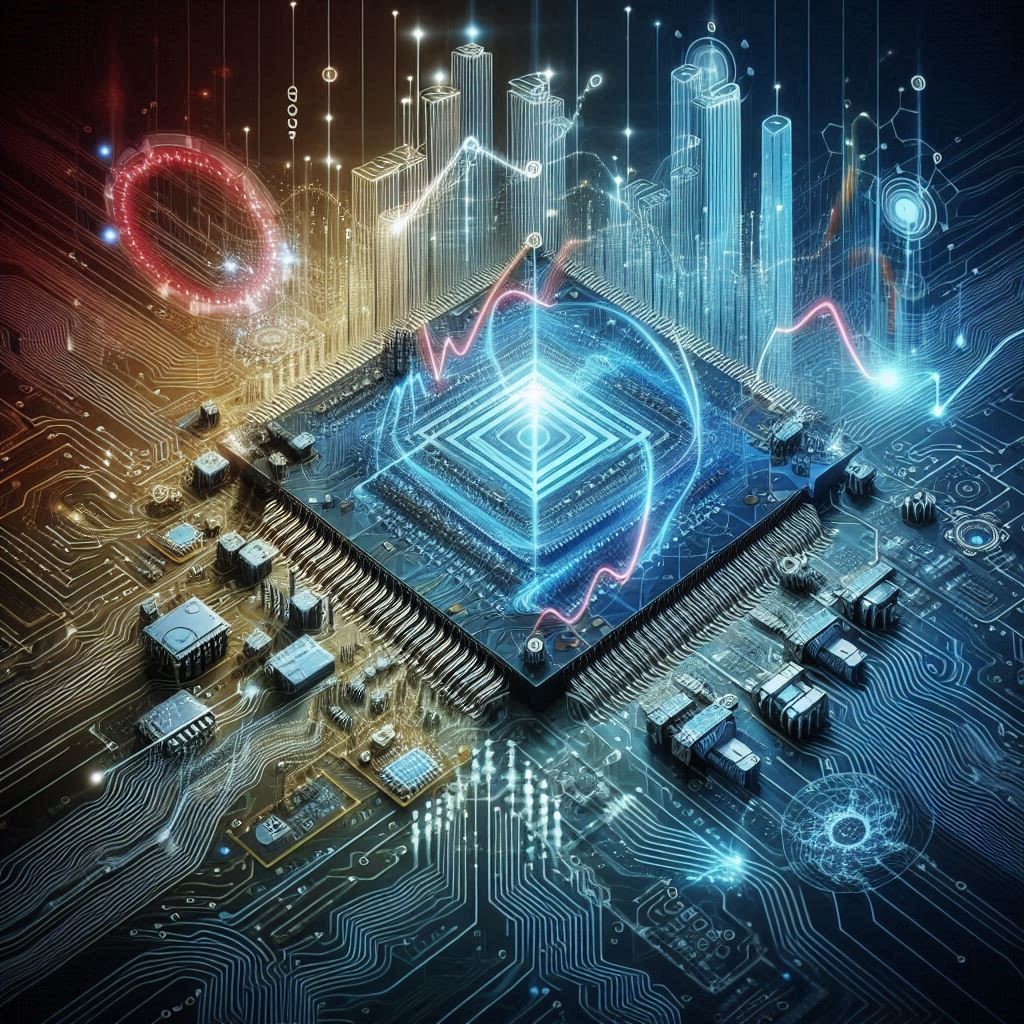
4. Neural Network Processors (NNPs)
As AI becomes more integrated into signal processing, Neural Network Processors (NNPs) are emerging as specialized DSPs that are optimized for running deep learning models and processing complex datasets. NNPs excel at handling large-scale neural networks, performing tasks such as image recognition, video processing, and real-time data interpretation.
Example: In autonomous vehicles, NNPs help process data from sensors, cameras, and LiDAR systems to detect obstacles, recognize road signs, and make driving decisions in real time.
Benefit: NNPs enable high-performance AI tasks such as image and video processing, boosting accuracy and efficiency in applications requiring real-time signal analysis.
5. 5G and Next-Generation Wireless Networks
The advent of 5G and next-generation wireless networks has significantly increased the demand for DSPs capable of handling higher-frequency signals, wider bandwidths, and low-latency requirements. DSPs play a crucial role in optimizing tasks such as beamforming, modulation, channel estimation, and interference mitigation in 5G and beyond.
With the expansion of 5G networks, DSPs are essential in supporting massive MIMO (multiple-input, multiple-output) technology and adaptive beamforming to optimize signal strength and data transmission efficiency.
Example: In 5G base stations, DSPs are responsible for handling high-frequency signals, ensuring reliable communication, and supporting high-speed data transfer with low latency.
Benefit: DSPs in 5G networks enhance signal processing capabilities, enabling faster, more reliable communication for applications like IoT, smart cities, and autonomous transportation.
6. Reconfigurable DSP Architectures
As the need for customized signal processing solutions grows, reconfigurable DSPs have become an important trend. These processors allow users to tailor their signal processing capabilities for specific applications using field-programmable gate arrays (FPGAs) and programmable DSPs. Reconfigurable DSPs provide greater flexibility and can be optimized for specialized tasks, which is crucial in industries like military and defense, medical imaging, and communications.
Example: In military applications, reconfigurable DSPs can be adjusted for different tasks such as radar signal processing, encryption, or satellite communication, depending on mission requirements.
Benefit: Reconfigurable DSPs offer greater adaptability and can be optimized for various signal processing tasks, making them ideal for applications requiring flexibility and performance.
7. High-Performance ADCs and DACs
As DSPs continue to handle more complex and high-fidelity signals, the demand for high-precision analog-to-digital converters (ADCs) and digital-to-analog converters (DACs) is increasing. These components are essential for ensuring the accurate conversion of real-world signals (like sound and images) into digital data that can be processed by DSPs.
High-resolution ADCs and DACs are now integrated with DSPs to ensure greater accuracy in signal processing. This is particularly critical in fields such as medical diagnostics, audio processing, and telecommunications.
Example: In medical imaging, high-performance ADCs and DACs are used in MRI machines and ultrasound systems to ensure high-quality image conversion for diagnosis.
Benefit: High-performance ADCs and DACs improve signal accuracy and enhance overall DSP performance, ensuring precise signal conversion in complex applications.
8. Low Power DSPs for Mobile and IoT Devices
With the growth of mobile devices, wearables, and IoT applications, power efficiency has become a crucial consideration. Low-power DSPs are emerging as a solution to handle complex signal processing tasks while minimizing energy consumption. Innovations in power management techniques, such as dynamic voltage scaling and energy-efficient architecture, are helping DSPs meet the growing demands of modern devices.
Example: In smartphones and fitness trackers, low-power DSPs process sensor data (like heart rate or GPS location) while optimizing battery life.
Benefit: Low-power DSPs extend battery life in mobile and IoT devices, enabling more efficient operation without compromising performance.
9. AI-Based Noise Reduction and Error Correction
Signal noise and errors are common challenges in signal processing, particularly in audio, telecommunications, and video applications. AI-powered DSPs are now being used to enhance noise reduction and error correction in real time. These DSPs analyze incoming signals, identify noise patterns, and apply filtering techniques to improve the clarity and accuracy of the processed data.
Example: In voice assistants, AI-enhanced DSPs eliminate background noise, improving speech recognition even in noisy environments.
Benefit: AI-based DSPs improve signal quality by automatically filtering out noise and correcting errors, leading to clearer and more accurate data processing.
10. 3D Audio and Spatial Sound Processing
With the rise of virtual reality (VR), augmented reality (AR), and gaming applications, the demand for immersive audio experiences has grown. 3D audio and spatial sound processing using DSPs are gaining traction as they enable a more realistic and dynamic auditory experience by simulating sound from different directions and distances.
Example: In VR headsets, DSPs create 3D audio that adapts to the user’s movement and position, enhancing the overall immersion.
Benefit: 3D audio processing using DSPs provides an enhanced, immersive sound experience that adds realism and depth to virtual environments.
A New Era for DSP Technology
The integration of these emerging technologies is revolutionizing the Digital Signal Processing (DSP) landscape. From AI and quantum computing to 5G networks and edge computing, these innovations are expanding the capabilities of DSPs, making them smarter, more efficient, and more versatile than ever before. As DSPs continue to evolve, they will play an even greater role in driving technological advancements across a wide array of industries, including healthcare, automotive, telecommunications, entertainment, and more. The future of DSPs is bright, and the possibilities are endless as they adapt to the growing demand for faster, more accurate, and real-time signal processing.
FAQ: Top Emerging Technologies in Digital Signal Processing (DSP)
1. What is Digital Signal Processing (DSP)?
Digital Signal Processing (DSP) involves the use of digital techniques to manipulate signals, such as sound, images, and sensor data, for a variety of applications. DSP systems convert analog signals into digital form, process them, and convert them back to analog if needed. Common uses include audio and speech recognition, image processing, and telecommunications.
2. How does AI enhance Digital Signal Processing?
AI enhances DSP by enabling processors to learn from data, adapt to new inputs, and improve performance over time. This integration allows DSP systems to handle complex tasks such as real-time speech recognition, object detection, and predictive analytics, making them smarter and more efficient.
3. What is edge computing in DSP?
Edge computing in DSP refers to processing data on local devices (at the “edge” of the network) rather than sending it to a centralized cloud for analysis. This reduces latency and bandwidth usage while enabling real-time decision-making, which is crucial in IoT, wearables, and autonomous vehicles.
4. How does quantum computing impact DSP?
Quantum computing can potentially revolutionize DSP by enabling the processing of large and complex datasets at unprecedented speeds. Quantum algorithms can perform certain signal processing tasks, such as Fourier transforms, much faster than classical DSP systems, significantly improving performance for high-demand applications.