Artificial Intelligence (AI) has made remarkable strides in recent years, influencing a wide range of industries, from healthcare and finance to manufacturing and transportation. One area where AI’s impact is particularly transformative is neuromorphic computing—a field dedicated to creating brain-inspired computing systems that emulate how the human brain processes information. Neuromorphic computing mimics the brain’s neural networks, offering energy-efficient, high-speed data processing capabilities that are ideal for a variety of applications, including artificial intelligence, robotics, autonomous vehicles, and smart devices.
In this article, we will explore how AI is driving the growth and evolution of the neuromorphic computing industry, the synergies between these two technologies, and what the future holds.
What is Neuromorphic Computing?
Neuromorphic computing is an interdisciplinary field that aims to design computer architectures based on the structure and function of the human brain. Unlike traditional computers, which process data sequentially, neuromorphic systems use parallel processing and event-driven communication to simulate brain-like computations. This allows for faster, more efficient processing with lower energy consumption, making neuromorphic systems ideal for AI applications that require real-time decision-making and learning.
Neuromorphic chips, such as Intel’s Loihi and IBM’s TrueNorth, are designed to perform cognitive tasks, such as pattern recognition, sensory processing, and learning, without needing large amounts of energy. This makes them highly efficient and well-suited for applications where both computational power and energy efficiency are critical.
The neuromorphic computing industry is expected to grow from USD 28.5 million in 2024 and is estimated to reach USD 1,325.2 million by 2030; it is expected to grow at a Compound Annual Growth Rate (CAGR) of 89.7% from 2024 to 2030. Growth in the neuromorphic computing industry is driven through the integration of neuromorphic computing in automotive and space operations. In space, where bandwidth is limited and the communication delay might be considered large, onboard processing capabilities are crucial.
AI’s Role in Advancing Neuromorphic Computing
AI is a catalyst for the rapid evolution of neuromorphic computing. Here are a few ways AI is accelerating the growth of this sector:
1. Enabling Smarter, Faster Decision-Making
AI’s ability to process large datasets and extract meaningful insights is complementary to the strengths of neuromorphic computing. By using neuromorphic systems, AI applications can make decisions in real time, much like the human brain. For instance, self-driving cars can leverage both AI and neuromorphic computing to make split-second decisions based on sensor data, without relying on cloud-based processing.
Neuromorphic computing allows AI systems to process sensory inputs—such as vision, sound, or touch—quickly and efficiently, making them ideal for applications like robotics, drones, and IoT devices, where real-time decision-making is crucial. With low-latency processing, AI models can operate seamlessly in environments that require constant, real-time updates.
2. Enhancing Machine Learning and AI Training
Machine learning algorithms are the backbone of AI applications, and their performance often relies on massive amounts of data and computational power. Neuromorphic computing offers a unique advantage here: it can process data in a distributed and parallel manner, similar to the brain’s neurons. This capability helps enhance the speed and efficiency of training machine learning models, particularly in edge computing environments where low energy consumption is essential.
Furthermore, neuromorphic systems can assist AI in continuous learning—a crucial aspect of machine learning. These systems are designed to adapt and self-optimize, making them more capable of improving over time, much like the way the brain learns from experiences. This makes them well-suited for online learning and adaptive algorithms.
3. Paving the Way for Cognitive Computing
The convergence of AI and neuromorphic computing is fostering the growth of cognitive computing—systems that simulate human-like thinking and reasoning. These systems are capable of learning, adapting, and making decisions based on context and experience, moving beyond traditional rule-based systems.
By combining AI’s ability to analyze data and recognize patterns with neuromorphic computing’s efficient, brain-like architecture, cognitive computing can be used in fields such as healthcare, where it can analyze medical data and assist doctors in diagnosing diseases, or in customer service, where it can improve natural language processing (NLP) to better understand and respond to human queries.
Download PDF Brochure @
https://www.marketsandmarkets.com/pdfdownloadNew.asp?id=227703024
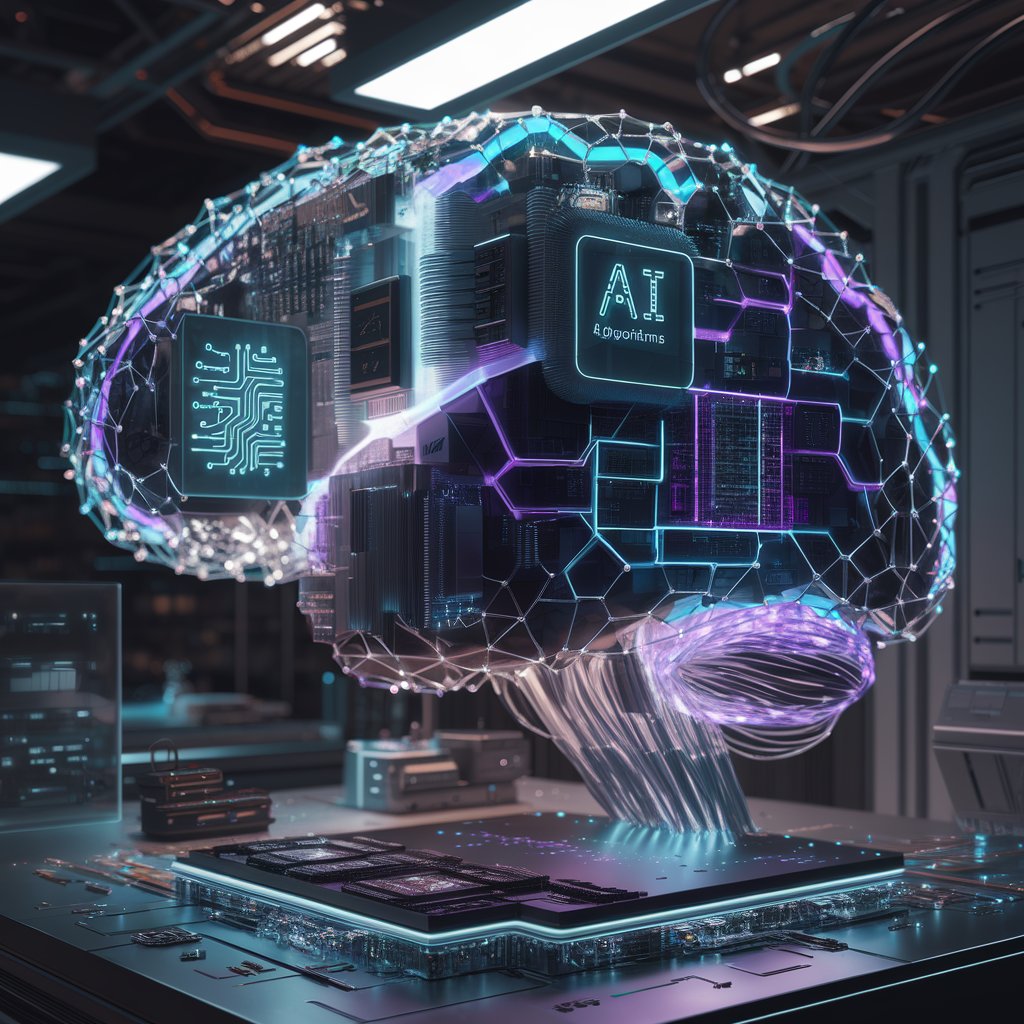
4. Revolutionizing Autonomous Systems
In the realm of autonomous systems, such as self-driving cars, drones, and robots, AI plays a central role in decision-making and navigation. Neuromorphic computing complements AI’s capabilities by providing a platform that processes sensory data in real time, allowing autonomous systems to react more quickly to changes in their environment.
For example, autonomous vehicles rely on a combination of AI and neuromorphic computing to analyze visual and auditory inputs from sensors (such as cameras, LiDAR, and radar) to navigate and avoid obstacles. Neuromorphic systems enable faster processing, reducing latency and enhancing the vehicle’s ability to make instantaneous decisions. This is critical in scenarios where safety is paramount.
5. Energy Efficiency for Edge Computing
A major advantage of neuromorphic computing is its ability to process data with minimal energy consumption compared to traditional computing systems. This is particularly important in edge computing, where devices need to process data locally rather than relying on cloud servers. With AI’s increasing presence in IoT devices, wearables, and smart homes, there’s a growing need for energy-efficient solutions that can perform complex computations without draining battery life.
Neuromorphic chips provide the energy efficiency needed for edge AI applications, making it possible to deploy AI-powered systems in remote or resource-constrained environments. From smart sensors to wearable health devices, neuromorphic computing allows for continuous, low-energy operation, which is essential for widespread AI adoption in everyday life.
The Future of AI and Neuromorphic Computing
As both AI and neuromorphic computing continue to evolve, their combined potential will drive significant innovation across industries. The future of AI and neuromorphic computing holds promise for even more powerful, efficient, and adaptable systems. Key developments to watch for include:
- Smarter AI systems that can learn and adapt in real-time, without needing large datasets or significant processing power.
- Increased integration of AI in autonomous systems where rapid decision-making, low power consumption, and real-time data processing are critical.
- Brain-machine interfaces that enable direct communication between AI systems and the human brain, potentially revolutionizing healthcare, prosthetics, and neuroscience.
- More widespread use of edge AI devices, powered by neuromorphic computing, that can operate autonomously and efficiently in remote locations or in real-time applications.
AI and neuromorphic computing are two of the most exciting technologies shaping the future of computing. AI’s ability to learn, adapt, and make decisions is amplified by neuromorphic computing’s brain-inspired architecture, leading to faster, more efficient processing with minimal energy consumption. As these two fields continue to evolve together, they will unlock new possibilities for autonomous systems, cognitive computing, and real-time AI applications, driving significant advancements across a wide range of industries.
For industries looking to harness the power of AI, integrating neuromorphic computing is becoming a key strategy to gain a competitive edge. As the technology matures, the combination of AI and neuromorphic computing will likely redefine the way we think about computing and intelligence itself.
Frequently Asked Questions (FAQ) – Impact of AI on Neuromorphic Computing Industry
1. What is Neuromorphic Computing? Neuromorphic computing refers to the design of computer systems inspired by the human brain’s architecture. It aims to replicate the brain’s neural networks, enabling parallel, energy-efficient, and real-time data processing. Neuromorphic systems are designed to process sensory data, perform cognitive tasks, and adapt to new information in a way that mimics human learning.
2. How does AI contribute to Neuromorphic Computing? AI contributes to neuromorphic computing by enhancing its capabilities for real-time decision-making, learning, and adapting. AI algorithms can leverage neuromorphic systems’ parallel processing and low-energy design to perform complex tasks, such as pattern recognition and autonomous decision-making, more efficiently than traditional systems.
3. What are the key advantages of Neuromorphic Computing? Neuromorphic computing offers several benefits, including:
- Energy Efficiency: Neuromorphic systems consume far less power than traditional computing systems, making them ideal for edge AI applications and mobile devices.
- Real-Time Processing: These systems process data instantly, providing low-latency response for applications like autonomous vehicles, robotics, and IoT devices.
- Adaptive Learning: Neuromorphic systems can continuously learn and adjust to new data, which is crucial for dynamic environments that require constant optimization, such as healthcare and autonomous systems.