The advent of artificial intelligence (AI) has revolutionized numerous sectors, with computer vision standing out as one of the most impactful applications. By enabling machines to interpret and understand visual information, AI in computer vision is transforming industries from healthcare to manufacturing. This article explores the key applications of AI in computer vision, highlighting its influence in healthcare, autonomous vehicles, surveillance, retail, and manufacturing.
The global AI in Computer Vision industry is expected to be valued at USD 17.2 Billion in 2023 and is projected to reach USD 45.7 Billion by 2028; it is expected to grow at a CAGR of 21.5% from 2023 to 2028.
Advancements in deep learning algorithms increased data availability, faster and cheaper computing power, and advancements in hardware technology like GPU and TPU are the driving factors of the AI in computer vision industry. Moreover, the market players are expected to benefit from the government’s initiatives aimed at supporting industrial automation and integrating AI into edge devices, creating profitable opportunities for them.
Artificial intelligence (AI) has made significant strides in various fields, with computer vision being one of the most transformative areas. By enabling machines to interpret and understand visual data, AI in computer vision is revolutionizing industries ranging from healthcare to autonomous vehicles. This article explores key technologies that are shaping the future of computer vision, including deep learning and neural networks, object detection and recognition, image segmentation, facial recognition, and edge AI.
1. Deep Learning and Neural Networks
At the heart of modern computer vision lies deep learning, a subset of machine learning that employs neural networks with multiple layers. These networks mimic the human brain’s structure and function, allowing computers to learn from vast amounts of visual data.
Key Advances:
Convolutional Neural Networks (CNNs): CNNs are particularly effective for image processing tasks. They automatically detect patterns and features within images, enabling systems to classify and analyze visual information with high accuracy.
Transfer Learning: This technique allows pre-trained models to be adapted for new tasks, significantly reducing the time and data required for training. By leveraging existing knowledge, organizations can implement computer vision solutions more efficiently.
Generative Adversarial Networks (GANs): GANs consist of two neural networks that work together to create new images from existing datasets. This technology has applications in generating synthetic data for training models and improving image quality.
Deep learning and neural networks have accelerated advancements in computer vision, making it possible to tackle complex visual recognition tasks with unprecedented accuracy.
2. Object Detection and Recognition
Object detection and recognition involve identifying and classifying objects within images or video frames. This technology is essential for applications ai in computer vision in various sectors, including security, retail, and autonomous vehicles.
Key Techniques:
Region-Based CNN (R-CNN): This approach combines CNNs with region proposal networks to detect objects within images accurately. It identifies potential object locations and classifies them, achieving state-of-the-art results.
YOLO (You Only Look Once): YOLO is a real-time object detection system that processes images in a single pass, making it highly efficient. Its ability to detect multiple objects simultaneously has made it popular for applications such as video surveillance and autonomous driving.
Single Shot Multibox Detector (SSD): SSD offers a balance between speed and accuracy, allowing for real-time detection of objects at various scales. This technique is widely used in applications requiring immediate feedback, such as robotic vision.
As object detection and recognition technologies advance, their applications are becoming increasingly diverse, enhancing safety, security, and efficiency in numerous industries.
Download PDF Brochure @
https://www.marketsandmarkets.com/pdfdownloadNew.asp?id=141658064
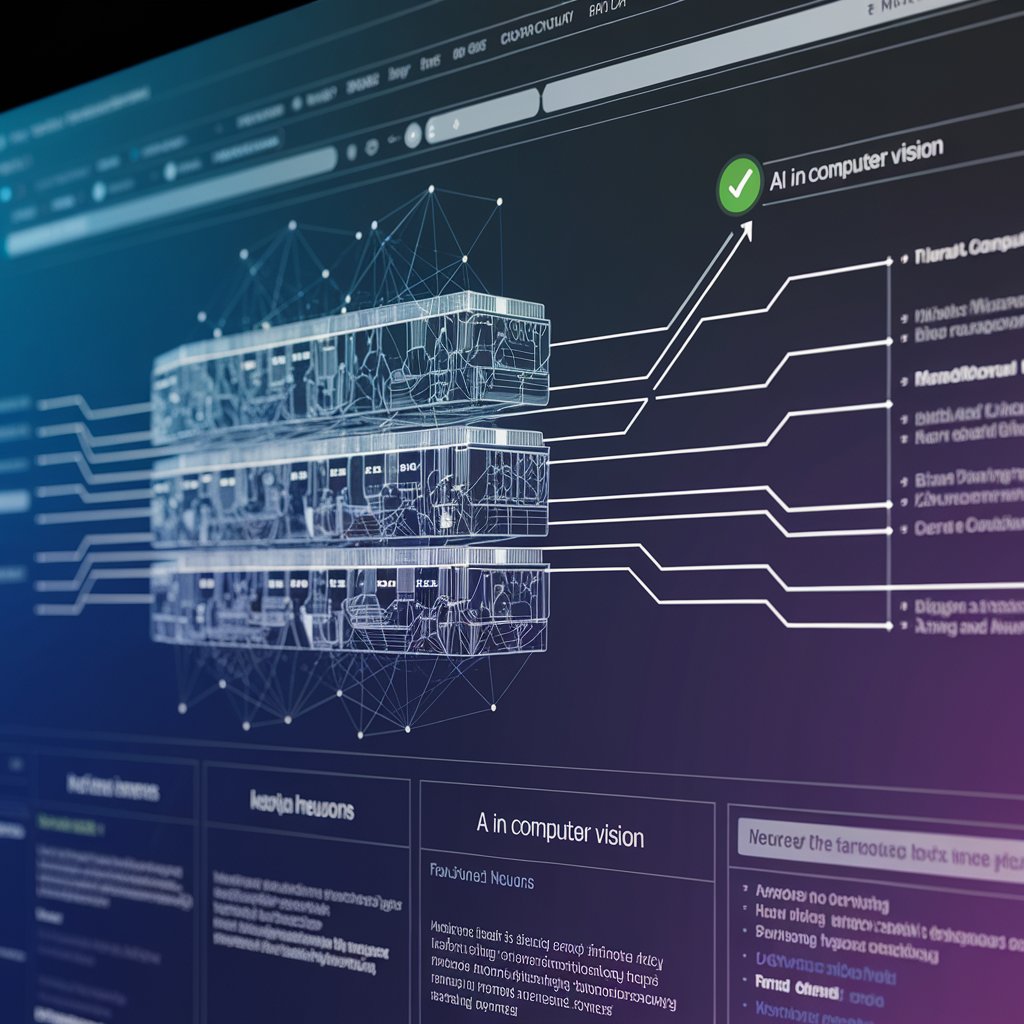
3. Image Segmentation
Image segmentation refers to the process of partitioning an image into distinct regions, facilitating more detailed analysis and understanding of the visual content. This technique is critical in applications such as medical imaging, autonomous navigation, and augmented reality.
Key Approaches:
Semantic Segmentation: This technique classifies each pixel in an image into predefined categories, providing a comprehensive understanding of the scene. It’s particularly useful in medical imaging, where precise identification of anatomical structures is crucial.
Instance Segmentation: Building on semantic segmentation, instance segmentation differentiates between individual objects within the same category. This capability is essential for applications like autonomous vehicles, where distinguishing between multiple pedestrians or vehicles is necessary.
Fully Convolutional Networks (FCNs): FCNs are specifically designed for pixel-wise predictions and are often used in image segmentation tasks. They leverage the strengths of CNNs while enabling detailed image analysis.
Image segmentation enhances the ability of AI systems to interpret visual data, paving the way for innovations in various fields, from healthcare diagnostics to smart city planning.
4. Facial Recognition
Facial recognition technology has gained prominence due to its applications in security, marketing, and user authentication. AI-driven computer vision systems can identify and verify individuals by analyzing facial features.
Key Technologies:
Feature Extraction: Algorithms identify key facial landmarks and features, such as the distance between eyes or the shape of the jawline. These features are then used to create a unique biometric profile for each individual.
Deep Learning Models: State-of-the-art facial recognition systems often employ deep learning techniques, such as CNNs, to improve accuracy and robustness. These models can learn to recognize faces even in challenging conditions, such as varying lighting or angles.
3D Face Recognition: This emerging technology uses 3D imaging techniques to capture the contours of a face, making it less susceptible to spoofing attempts and improving recognition accuracy.
Facial recognition technology continues to evolve, raising important discussions about privacy and ethics while offering significant benefits in security and user experience.
5. Edge AI for Computer Vision
Edge AI refers to the deployment of AI algorithms directly on devices at the edge of the network, rather than relying solely on centralized cloud processing. This approach is particularly beneficial for computer vision applications that require real-time analysis and low latency.
Advantages:
Reduced Latency: Processing data on-device minimizes delays, making it ideal for applications such as autonomous vehicles and real-time surveillance systems where split-second decisions are critical.
Bandwidth Efficiency: Edge AI reduces the need to transmit large volumes of data to the cloud, conserving bandwidth and enhancing privacy by keeping sensitive data local.
Enhanced Reliability: Local processing ensures that systems remain operational even in low-connectivity environments, increasing resilience for applications in remote areas or during network disruptions.
The integration of edge AI into computer vision systems is driving innovation across various sectors, enabling more responsive and efficient applications.
AI technologies are fundamentally transforming the field of computer vision, driving advancements in deep learning, object detection, image segmentation, facial recognition, and edge AI. These innovations AI in computer vision are not only enhancing the accuracy and efficiency of visual analysis but are also paving the way for new applications across diverse industries. As the capabilities of future AI in computer vision continue to expand, we can expect to see even more groundbreaking developments that will shape the future of technology and its impact on our daily lives.