Video surveillance has long been a cornerstone of security systems, used to monitor and safeguard people, properties, and assets. Traditionally, video surveillance systems relied on human operators to analyze footage in real-time or review recorded material. However, with the rapid advancement of Artificial Intelligence (AI) technologies, video surveillance has evolved into a more intelligent, automated, and efficient tool, capable of providing deeper insights and faster responses. Today, technologies such as Machine Learning (ML), Deep Learning (DL), Generative AI (GenAI), Computer Vision (CV), and Natural Language Processing (NLP) are reshaping video surveillance by automating analysis, improving accuracy, and enabling more sophisticated functionalities.
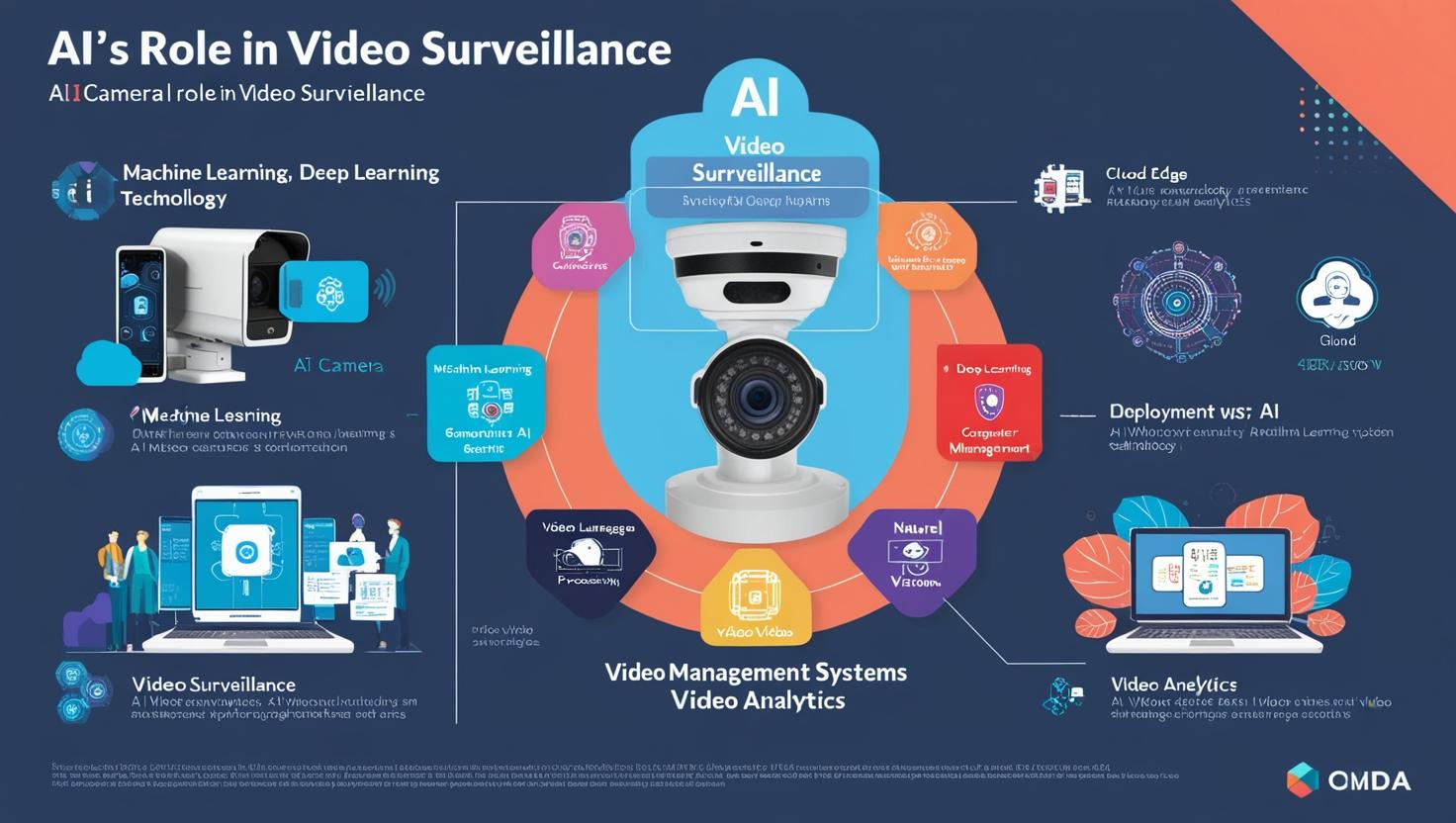
This article explores how these AI in Video Surveillance by Technology are being integrated into modern video surveillance systems and their significant impact on security and monitoring across various industries.
1. Machine Learning (ML) in Video Surveillance
Machine Learning (ML) involves algorithms that allow systems to learn from data, identify patterns, and make decisions without explicit programming. In video surveillance, ML enhances the system’s ability to recognize behaviors, movements, and anomalies by processing large volumes of video footage quickly and efficiently.
Key Applications of ML in Video Surveillance:
- Anomaly Detection: ML models can be trained to detect unusual behaviors such as loitering, theft, or vandalism. The system learns from past data and continuously improves its detection capabilities.
- Person and Vehicle Tracking: ML algorithms can identify and track individuals or vehicles across multiple cameras, enhancing the system’s ability to follow subjects through a facility or public space.
- Pattern Recognition: ML can recognize specific patterns of activity, such as crowd formation, unusual movement in restricted areas, or specific actions like people entering off-limits zones.
By continuously learning from new footage, ML-powered video surveillance systems get smarter over time, reducing false positives and increasing the efficiency of security monitoring.
2. Deep Learning (DL) in Video Surveillance
Deep Learning (DL), a subset of machine learning, utilizes neural networks with many layers (also known as deep neural networks) to analyze and interpret complex data. Unlike traditional ML models, deep learning can process and analyze raw video data, enabling much more sophisticated video surveillance capabilities.
Key Applications of DL in Video Surveillance:
- Facial Recognition: Deep learning-based systems are highly effective in identifying individuals based on their facial features. By analyzing vast datasets, these systems can match faces in real-time with databases, increasing accuracy and efficiency in identifying people within secure environments.
- Object Detection and Classification: DL algorithms are widely used for recognizing and classifying objects in video feeds, such as bags, vehicles, or equipment. This is useful in scenarios where specific objects need to be detected, like unattended bags in airports or restricted vehicles in a no-parking area.
- Action Recognition: DL can be used to detect specific actions or activities within video footage, such as aggressive behavior, fighting, or illegal activity. These insights help security personnel take quicker, more informed actions.
Deep learning’s ability to learn hierarchical patterns in data and handle unstructured inputs like video makes it a powerful tool for advanced surveillance systems.
3. Generative AI (GenAI) in Video Surveillance
Generative AI (GenAI) refers to algorithms capable of generating new content from learned data. While still an emerging field in video surveillance, GenAI has enormous potential to enhance the functionality of security systems by generating synthetic data, enriching surveillance footage, and enabling predictive capabilities.
Key Applications of GenAI in Video Surveillance:
- Synthetic Video Generation: GenAI can generate synthetic training data, which is particularly useful when training machine learning models for rare events or hard-to-reproduce scenarios. This synthetic data can supplement real-world footage to improve the accuracy of surveillance systems.
- Predictive Analytics: By analyzing historical video data, GenAI can be used to predict potential security threats or patterns of behavior before they occur. For example, it could forecast when and where suspicious activity is likely to happen, giving security personnel a head start in preventing incidents.
- Enhancing Low-Quality Footage: GenAI can be used to enhance the quality of low-resolution video footage, making it easier to identify details that might otherwise be missed. This feature can significantly improve the value of surveillance in areas where video quality is often compromised, such as in low-light conditions.
As GenAI technology matures, its ability to enhance surveillance data and improve predictive capabilities will be integral to the future of security.
Download PDF Brochure @ https://www.marketsandmarkets.com/pdfdownloadNew.asp?id=84216922
4. Computer Vision (CV) in Video Surveillance
Computer Vision (CV) refers to the ability of AI systems to interpret and understand visual data. In video surveillance, computer vision plays a pivotal role in extracting meaningful information from video feeds and improving situational awareness in real-time.
Key Applications of CV in Video Surveillance:
- Real-Time Monitoring and Alerts: CV algorithms can continuously monitor video footage and automatically detect key events, such as movement or people entering restricted areas, providing immediate alerts to security teams. This reduces the need for manual monitoring and allows for faster responses to incidents.
- License Plate Recognition (LPR): Computer vision is commonly used in vehicle surveillance to capture and read license plates. LPR systems are employed in parking lots, toll booths, and border control for real-time tracking of vehicles.
- Crowd Monitoring: CV can be used to monitor crowd density and detect dangerous crowd behavior, such as stampedes or large gatherings in areas not designed for crowds. This is particularly valuable in public safety and large event management.
By giving machines the ability to “see” and “understand” visual data, computer vision significantly enhances the power of video surveillance systems.
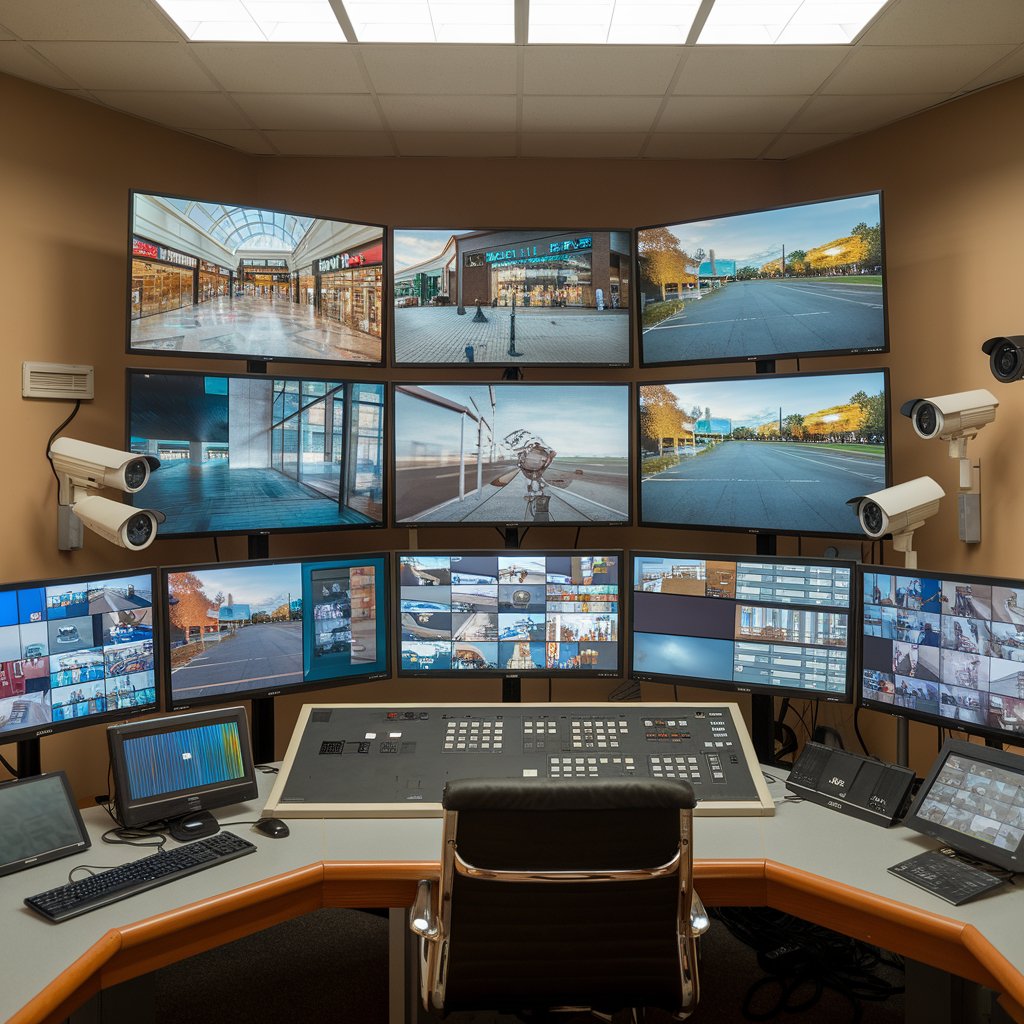
5. Natural Language Processing (NLP) in Video Surveillance
Natural Language Processing (NLP) is a branch of AI that allows computers to understand and interpret human language. In the context of video surveillance, NLP enables systems to interact with security teams in a more intuitive and effective manner.
Key Applications of NLP in Video Surveillance:
- Voice-Activated Commands: Security systems can be enhanced with voice recognition and NLP capabilities, allowing security personnel to issue commands or retrieve specific footage via voice, improving operational efficiency.
- Automatic Report Generation: NLP can be used to transcribe and summarize security events. By analyzing video metadata, the system can generate detailed reports, such as summarizing the key incidents or security alerts, saving time for security analysts.
- Real-Time Communication: NLP algorithms can process spoken language in surveillance situations, such as conversations between individuals, enabling systems to detect potential threats based on verbal cues (e.g., aggressive tone or keywords related to suspicious activities).
Integrating NLP with video surveillance systems creates a more seamless and interactive experience for security teams, enhancing their ability to respond to and analyze incidents.
The Future of AI in Video Surveillance
As AI technologies continue to evolve, the potential for AI in video surveillance will only grow. The integration of 5G networks, edge computing, and IoT devices will provide even faster data processing, allowing AI-powered surveillance systems to respond in real-time to emerging threats. Moreover, combining AI with cloud computing will allow for enhanced data storage, sharing, and analytics, making surveillance data more accessible and actionable than ever before.
AI-powered video surveillance, driven by technologies such as Machine Learning, Deep Learning, Generative AI, Computer Vision, and Natural Language Processing, is redefining how security systems operate. These technologies enable real-time analysis, predictive capabilities, and smarter decision-making, leading to more effective and efficient security operations. As AI continues to mature, the integration of these technologies will not only enhance surveillance capabilities but also create safer environments for businesses, individuals, and communities. The future of video surveillance is undoubtedly intelligent, proactive, and data-driven, providing unprecedented insights and responding to security challenges faster than ever before.